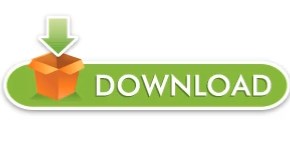
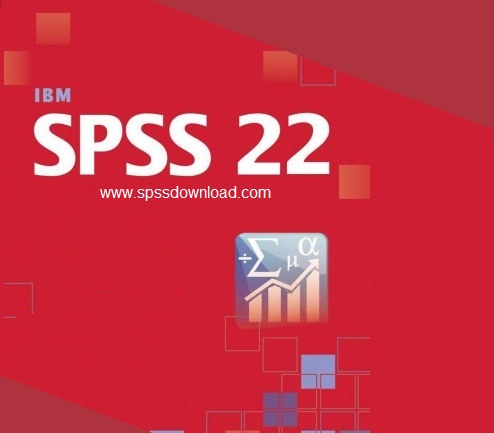
Likewise we use the test command to get the test on mainįinally, we use the test command to test the interaction of of collcat by mealcat. We use the test command to test the two terms associated with collcat to get the main effect of collcat. Then we use the regression procedure based on those variables. Below weįirst create simple regression coding for both variables collcat and mealcat. We can do these same analyses using the regress command. We can show a graph of the adjusted means as shown below. The option emmeans (which stands for Estimated Marginal Means) gives the adjusted means broken down by collcat and mealcat The results show a main effect of collcat (F=4.5, p-0.0117), a main effect of mealcat (F=509.04, p=0.0000) and an interaction of collcat by mealcat, (F=6.63, p=0.0000). One traditional way to analyze this would be to perform a 3 by 3 factorial analysis of variance using the We drop the label for mealcat because this can get in the wayĪt some of the points we will be demonstrating. means tables= api00 by mealcat by collcat Look at the three variables we are interested in. We will first input the elemapi2 data file and have a quick We will see that there is an interaction of these categorical variables, and will focus on different ways of further Performance in the school, and we will look at the interaction of these two categorical variables as well. In this chapter we will look at how these two categorical variables are related to api In other words, we think that there might be an interaction of these twoĬategorical variables. Possible that the impact of mealcat might depend on the level of collcat. Mealcat and collcat may be related to api00, but it is also Thought of as the number of parents with some college education, and we could think of it as being broken up into
SPSS 23 STOP ANALYSIS FREE
We could think of mealcat as being the number of students receiving free meals and broken up The variable mealcat is the variable mealsīroken up into 3 categories, and the variable collcat is the variable some_col broken intoģ categories. (performance on the api in the year 2000. We will focus on the variables mealcat, and collcat as they relate to the outcome Simple effects of mealcat at levels of yr_rndįor this chapter we will use the elemapi2 data file that we have been using in prior chapters. Simple effects of yr_rnd at levels of mealcatĦ.8.2 Example 2. Analysis with 2 categorical variablesĦ.2.1 Analyzing Simple Effects Using MANOVA and GLMĦ.2.2 Analyzing Simple Effects Using REGRESSIONĦ.3.1 Analyzing Simple Comparisons Using MANOVA and GLMĦ.3.2 Analyzing Simple Comparisons Using REGRESSIONĦ.4.1 Analyzing partial interactions Using MANOVA and GLMĦ.4.2 Analyzing partial interactions Using REGRESSIONĦ.5.1 Analyzing interaction contrasts using MANOVA and GLMĦ.5.2 Analyzing interaction contrasts using REGRESSIONĦ.6.1 Computing Adjusted Means via MANOVA and GLMĦ.7 More Details on Meaning of the CoefficientsĦ.8 Simple Effects via Dummy Coding vs.
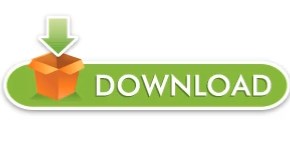